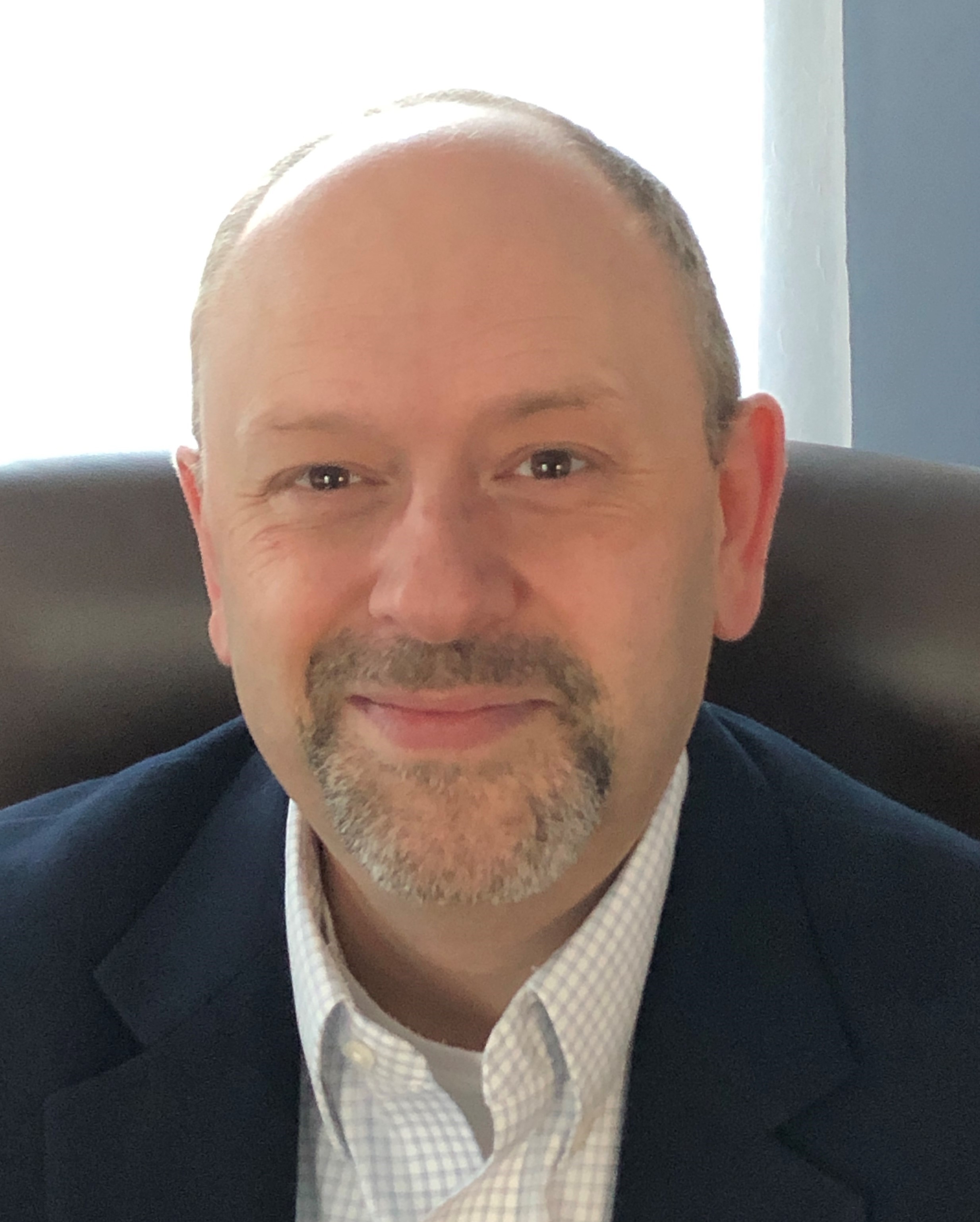
Artificial intelligence/machine learning (AI/ML) seems to be presented as the solution to absolutely every problem in our lives lately. How do we get from today to this projected future state? It is worth remembering that the routine use of Robotic Process Automation (RPA) and AI/ML across multiple industries, including pharmacovigilance, is not new. Indeed, Uppsala Monitoring Centre has and continues to lead on pioneering machine learning work dating back to the first routine use of machine learning in pharmacovigilance on duplicate report detection. There is always promise and excitement within pharmacovigilance to be able to leverage technological advances. Currently, technologies are being increasingly trusted to reduce the cost of current activities and to potentially improve how patient benefit-risk is assessed.
It is important, however, to note that pharmacovigilance activities in a pharmaceutical company must adhere to a diverse set of legal regulations that dictate how activities are conducted across the entire pharmacovigilance cycle. These regulatory frameworks are themselves complex and made further complicated by the many variations that exist worldwide. Pharmaceutical company processes align around these diverse regulations to ensure the integrity of pharmacovigilance activities. It is these activities, which are essential to ensuring patient safety, maintaining trust by the public in medicines and vaccines, and for maintaining trust in AI/ML tools by all stakeholders, where global harmonisation is important to reduce unnecessary effort and maintain focus on tasks benefiting patient safety.
Ensuring trust today
It is accepted practice to examine incoming and outgoing datasets, examine the automation software, potentially through a line-by-line code review, monitor automation metrics, and utilise risk management and control plans to identify potential areas for failure and resolutions. This RPA documentation set, along with the RPA code itself, is made available for internal audit and external inspection. This approach essentially treats RPA as a “white box” entity, where every component of the technology is open and available for review, giving full transparency. Does such a management model fit given recent exciting advances with AI/ML?
Currently, one industry wide struggle being grappled with in regulated areas is how to responsibly govern AI/ML software and algorithms. A recent Accenture industry survey found that 92% of biopharma executives note the need to have more systematic ways to manage emerging technologies responsibly. Should there be an expectation that lines of algorithm code are inspected or reviewed as with RPA technologies, or should we ignore the algorithm details and only inspect the results? Should every dataset used to validate an algorithm be retained as evidence? What role should pre-trained large language models (LLMs) or generative AI (GenAI) play within pharmacovigilance when the training datasets and algorithm specifics are unknown? How do we handle possible AI hallucinations and the variability of generated results?
Rethinking trust
Some current thinking on AI/ML implementation appears to be headed in the same direction as for RPAs and other technologies. However, we believe that this will not be sustainable in the future. Already, AI/ML algorithm software and pre-trained models are rapidly increasing in complexity, the ability to store ever larger test datasets and results is becoming more difficult, and the move to federated networks like Sentinel in the US and Darwin in Europe where data is available for analysis but not centralised makes it difficult to retrieve that data.
To ensure appropriate use of the technology, assessment of AI/ML outputs and related processes is arguably growing in importance given the increasing complexity of AI/ML and the potential use of LLMs. We suggest that ensuring trust in AI/ML technology can make use of existing risk-based pharmacovigilance processes as a framework. The future of trust in AI/ML could focus on monitoring the outcomes of a process for safety, reliability, and effectiveness. Using a risk-based approach could help ensure that any change in or impact to business processes is fully understood and can be successfully managed. Moreover, it should involve all stakeholders working closely together to harmonise on a process for implementing and monitoring AI/ML. This way, responsible data access to all partners in the pharmacovigilance ecosystem will be assured.
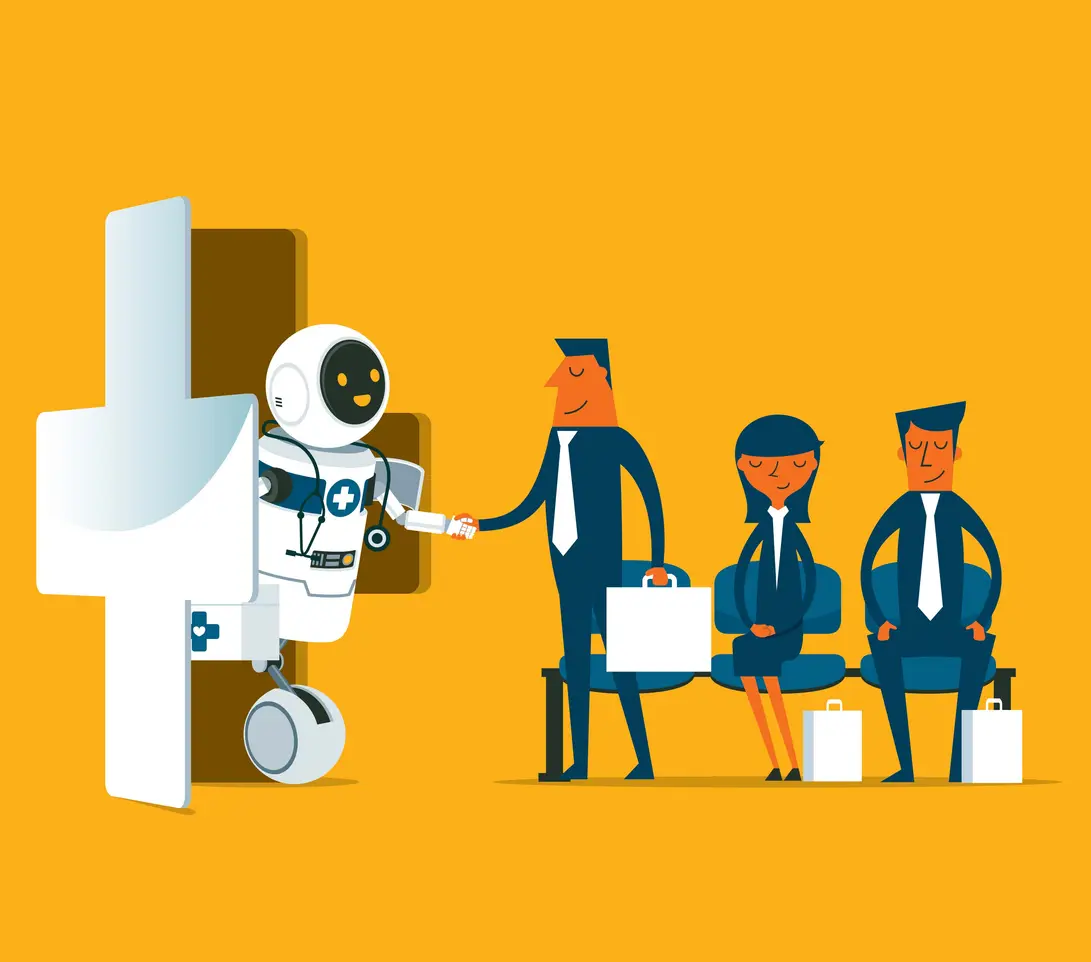
Risk based approach to ensuring trust in AI/ML
By utilising a modified and simplified version of existing pharmacovigilance system frameworks, and focusing on the safety, reliability, and effectiveness of the output of AI/ML systems rather than the dataset and algorithm itself, we may build trust in AI/ML much like we do for existing technologies. Building trust ensures that when a pharmaceutical company takes a systematic approach to assess and monitor the quality of data inputs and outputs, with targeted spot checking that is proportional to risk, a pharmacovigilance organisation can successfully balance the goals of ensuring patient safety while making the most use of the advantages of an AI/ML system. This approach does not require access to algorithms or datasets. Ensuring trust rather can rely on a “black box” approach to AI/ML technologies that focuses more on outcome validation and access to high-level metadata.
Harmonise global regulations
Ensuring trust in AI/ML systems is one example of an area in which all participants must engage each other and constructively come to a set of agreements. Continuing the current “white box” mindset will stifle innovation and limit advances that may benefit patients, particularly as the volume of pharmacovigilance data and myriad of data sources continue to increase at a rapid pace.
The conversation must result in a unified set of global regulations around AI/ML systems. A scenario like the disparity of adverse event reporting rules between countries must not be allowed to propagate into regulations for AI/ML systems. If global regulations remain diverse and unaligned, delays or increased risk may result; this will present a barrier to pharmaceutical companies utilising, implementing, and reaping the benefits from new technological advances.
Studies have shown that AI/ML innovations are starting to make progress within pharmacovigilance, so it is important to be nimble, react quickly, and strive to not stifle innovation. As part of the AI/ML conversation, global forums should be organised to discuss and collaborate. Trancelerate’s important work as a cross-industry consortium on intelligent automation validation and guidance documents is an example of such a forum. Making sure that recommendations for use of AI/ML in pharmacovigilance are suitable for all stakeholders is critical; in this regard, we view the CIOMS working group on AI as a progressive step forward.
Ultimately, patient safety remains the focus
Patient safety remains the unwavering focus of all pharmacovigilance activities. AI/ML systems offer great promise in improving operational processes to free human resources for higher-value activities and providing insights that might not be possible otherwise. By working together to rethink and harmonise the global regulatory framework, and by focusing on technological outputs rather than the technology itself, the potential of AI/ML can be unlocked to enable advances in better understanding the benefit-risk of medicines and vaccines for patients without compromising on patient safety.
Conflict of interest: All authors are employees of GSK and hold stock and/or stock options. Andrew Bate previously led the research function at UMC until 2009.
Disclaimer: Any opinions expressed in articles published in Uppsala Reports are those of the authors named and, unless otherwise noted, do not necessarily reflect the views of the Uppsala Monitoring Centre.
Read more:
“Technology Vision 2023 for Biopharma”, Accenture, 2023.
H Wang, Y.J. Ding, Y Luo, “Future of ChatGPT in Pharmacovigilance”, Drug Safety, 2023.
JU Stegmann, et al, “Trustworthy AI for safe medicines”, Nature Reviews Drug Discovery, 2023.