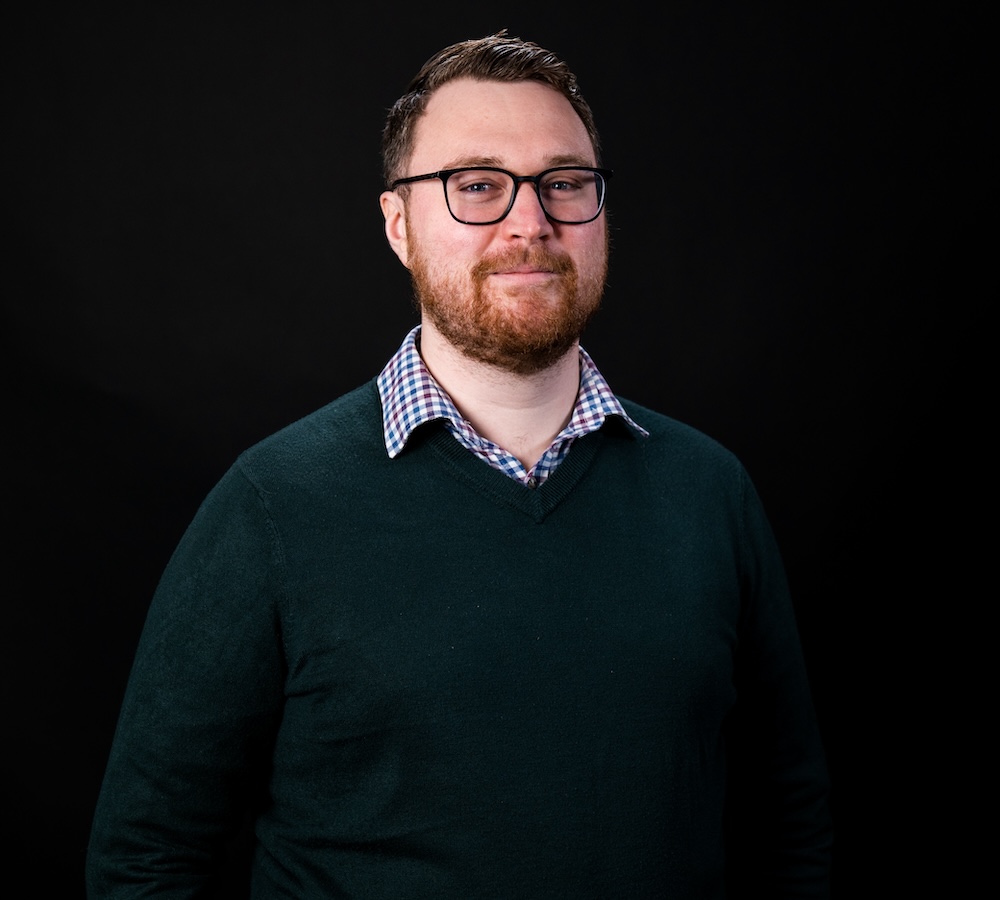
VigiBase is fast approaching 40 million reports of adverse events following drugs and vaccines, with no indication of slowing down its growth. So far, in 2024, VigiBase has received on average about 50 000 new reports per week. The sheer size of VigiBase makes it an amazing resource for pharmacovigilance; however, a natural consequence of this high rate of reporting is that we can sometimes get more than one report in VigiBase about the same adverse event in the same patient. There are many ways this can happen. Sometimes, there are multiple reporters of the same event or a single patient may report to multiple places. Another is that follow up information can be mistakenly unlinked to the original report.
Duplicate reports pose several problems for pharmacovigilance. A key example arises when doing statistical signal detection, which is when we try to identify the adverse events which are happening more frequently in combination with a drug than we would otherwise expect to see by chance. Imagine we have some adverse event reports for a drug that specify experiencing a headache. Given the background rates on reporting of headache, we would expect 10 of the reports to mention headache by chance. Then imagine that for each of the patients who experienced a headache, VigiBase had received two independent reports of their adverse event. Suddenly, this combination looks like it's happening twice as often as we would expect. This might lead us to investigate the combination as a potential safety signal, wasting valuable time that could be spent investigating other potential signals. Clearly, it would be better to remove duplicate reports from the database before we do our statistical analyses.
For VigiBase, this task is impossible to do manually due to the large number of reports it receives daily. So, it becomes necessary to come up with an algorithm to do it for us. This is a more challenging problem than it sounds. Just because two reports are duplicates of one another, doesn't mean that they look identical. Different reports might use different terms to describe the same adverse event, or they might include more or less information about the patient. Conversely, two reports may not contain enough information to reliably decide whether they are duplicate reports or not.
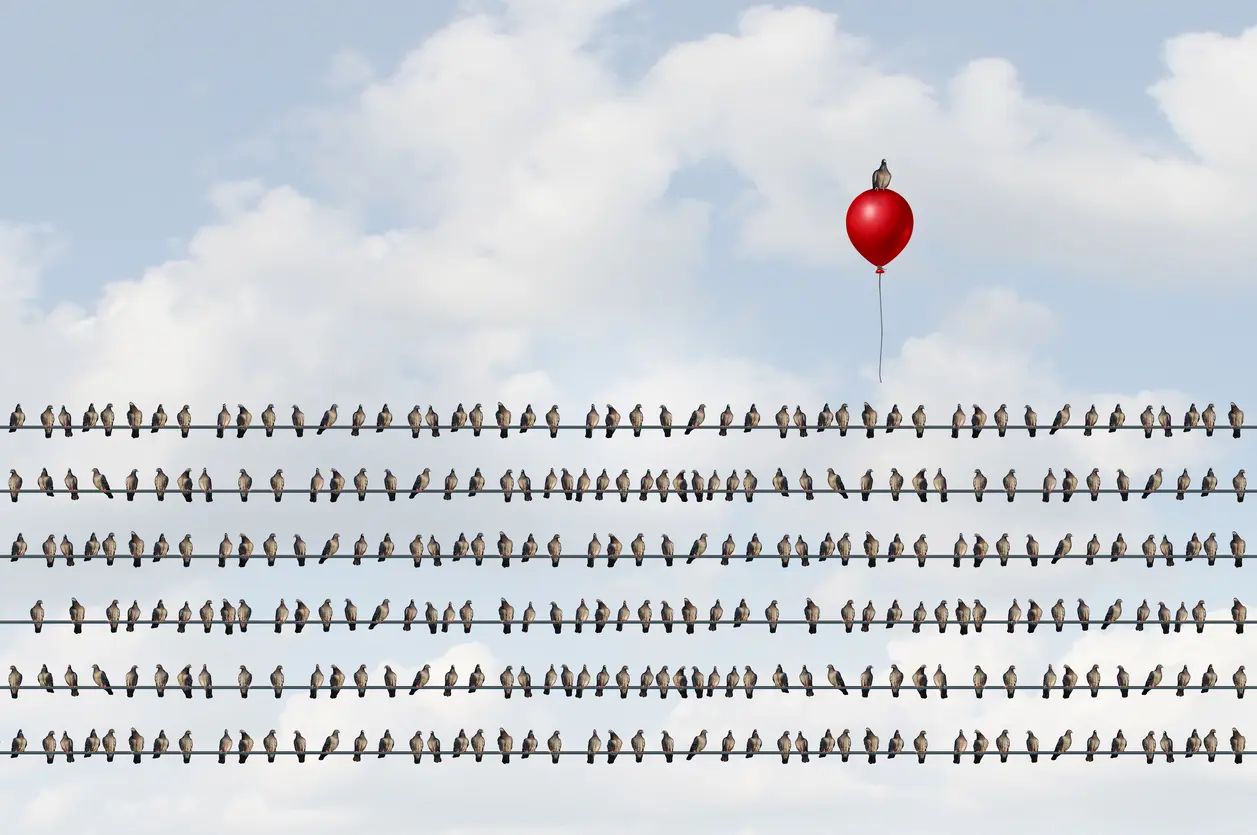
Previous efforts to detect duplicates have focused on probabilities, comparing the likelihood of specific combinations of drugs, reactions, sexes, ages etc. occurring on a given pair of reports based on background reporting rates derived from VigiBase. If it seems too unlikely to have occurred by chance, then we suspect they're duplicates. This approach has been used with success at Uppsala Monitoring Centre (UMC) for several years. However, methods like these can run into problems, especially in databases as large and diverse as VigiBase. One place where previous approaches are known to perform poorly is with reports of adverse events following vaccinations. Consider the vaccine against human papillomavirus (HPV). Most vaccine recipients are going to be girls of around the same age, with many patients being vaccinated on the same day. If you have two HPV vaccine reports, and both report the same sex, age, date of vaccination and adverse event, this may still not be sufficient evidence to suspect them of being duplicates. These challenges have made duplicate identification among vaccine reports unreliable.
Over the past two years, researchers at UMC have been working on a new algorithm for duplicate detection for both drug and vaccine reports. It builds upon the strengths of earlier approaches, but also implements new methods comparing pairs of reports. For example, we use a new way of capturing any date information mentioned on the report, from drug administration periods to the start and end date of the reaction, to the free text narrative. We use this date information to determine whether the timelines described in the reports are compatible. If they are, the reports are more likely to be duplicates, if they aren’t, then they may be separate reports. The method also uses machine learning to understand how to effectively weigh evidence from different parts of the reports to decide whether to suspect a pair as being duplicates. In all our tests, this new approach works as well as or better than previous approaches for both drugs and vaccines.
Effective duplicate detection is just one cog in the machine of pharmacovigilance, but once the new method is in place, pharmacovigilance practitioners worldwide will have a sharper tool to find true safety signals, ultimately improving patient safety.