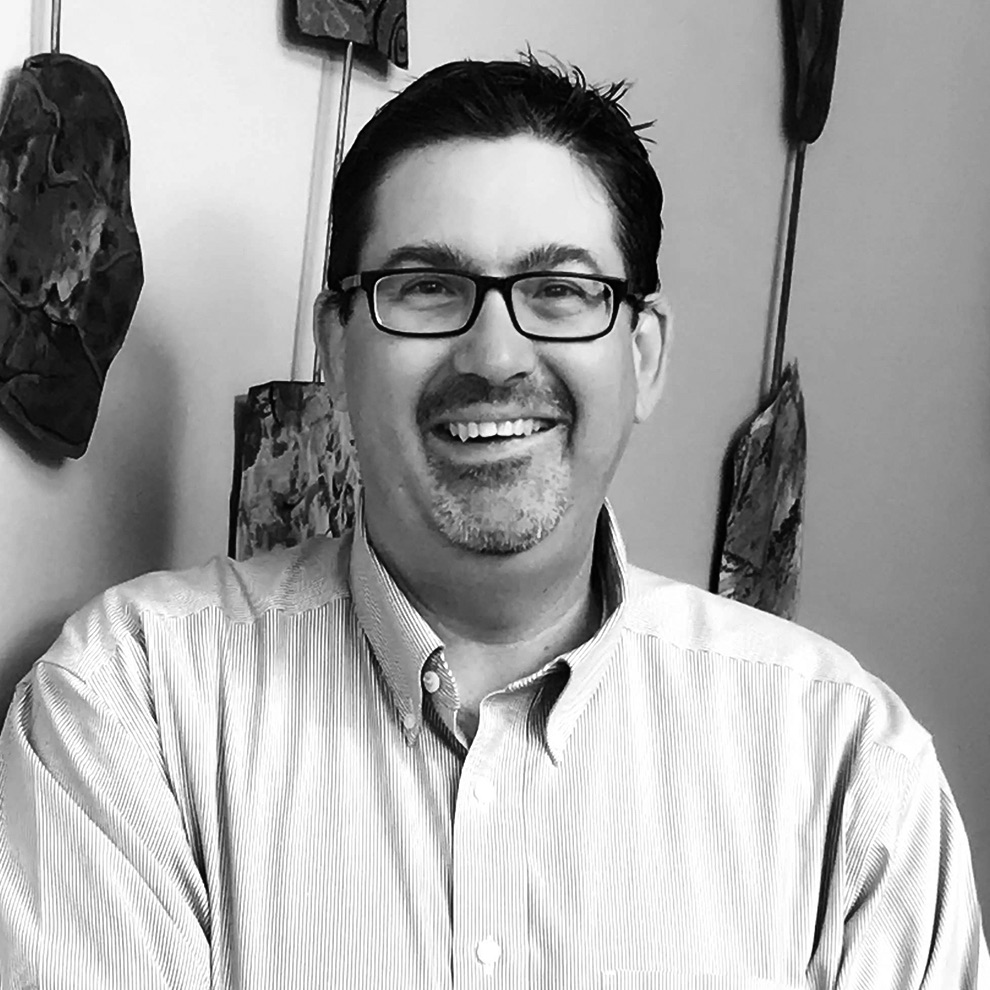
In a few years, some pharmacovigilance activities may become fully automated. Contract research organisations, business process outsourcing and technology providers will all be able to use artificial intelligence (AI) to do the work that once only humans could do. As appealing as this may sound, businesses need to use caution before totally immersing themselves in AI. If quality is
not maintained, companies will be trading increased efficiency for reduced quality. The best strategy for implementing AI is to enhance – not replace – current work processes. Rather than stand-alone solutions, AI and automation should be considered tools that add value, while lowering cost without sacrificing quality.
Two pharmacovigilance processes that would benefit most from automation are intake (the processing of incoming safety reports) and triage (the assessment of a case’s seriousness). Automation of these early-stage procedures will have the maximum impact downstream. By digitising data early in the workflow, AI can then be added through other processes, such as follow-up querying and narrative generation.
Even so, we must be prepared for the challenges that real-world data acquisition can represent. Ideally, data would be entered electronically in an intake system once, and then sent directly to the safety database. The reality is quite different, however. Often data is not readily available in easy-to-use electronic formats. It could be submitted as multiple reports emailed in a single spreadsheet, or as information collected via a patient reporting hotline.
What has been described so far may not yet sound exactly like AI, but without turning raw input into electronically consumable data, it will be challenging to implement AI technology. Once electronic data is available, machines can be trained to do more complex tasks, such as determining the seriousness of a case. At that point in the process, it is paramount that the quality of the data the machine is training on is of the highest standard. No matter how good the AI algorithm is, if the data is not good, the machine will learn the wrong things.
Though some will push toward a purely touchless process with no human intervention, it would be prudent to take a more strategic approach to processing cases. Some of that could include a risk-based methodology, in which serious and non-serious cases are processed differently. One should also consider adding sampling methodology where, for example, 10% of cases read by the machine are sent back to a human for peer quality control on how the case was processed. By feeding both positive and negative feedback, the program should be able to learn and self-correct over time. With this approach, quality can be maintained and standards improved in the long run.
There will be rapid advances during the next few years. As a result, it will be crucial to differentiate between companies that truly offer AI and automation, versus those that simply claim to do so. It will be important to understand the quality of the data used to build the models, and to know how those models will continue to learn. The experience and knowledge of those creating the training data that the models are built from will become a critical factor. There is an opportunity for those with technical acumen and process excellence to engage in partnerships that meet industry needs, and offer a unique service that exhibits both efficiency and high quality.