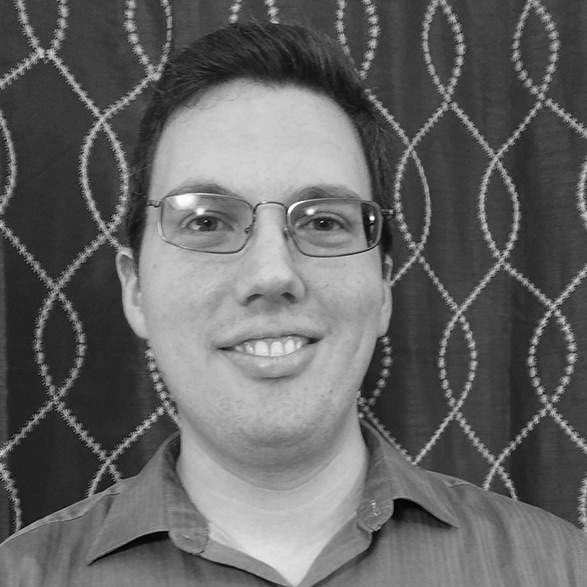
Spontaneous reporting systems are one of the best means of detecting rare adverse effects related to the use of medical products. The US Food and Drug Administration (FDA) monitors product safety issues in part by employing an automated tool to flag product-adverse effect pairs that are reported more commonly together than expected. This method may report a false association if there are duplicates of some cases in the data. Even a handful of duplicates can appear as a surge in reporting that triggers an alert and requires time and manual investigation to debunk.
Duplicate cases can arrive in reporting systems in several ways, but often arise as a side effect of mandatory reporting rules intended to enhance safety. Manufacturers must submit case reports involving their products to the FDA. However, each manufacturer may submit their own independent report for the same patient if that patient was exposed to multiple medical products, thus increasing the chances of duplicates ending up in the system. Without an efficient means of filtering these duplicate cases, performing accurate aggregate case analyses requires significant manual effort.
Along with our reviewer colleagues, we recently published the details and evaluation results of an algorithm, now incorporated into our Decision Support Environment platform, that was designed to assist FDA medical reviewers and epidemiologists in quickly identifying duplicate cases. The algorithm is based on the probabilistic Fellegi-Sunter model of record linkage, which compares two records on a number of fields and attempts to determine the likelihood that they describe the same incident.
The most novel aspect of the algorithm was the use of free text from case report narratives. Previous efforts have focused mainly on structured information – like age, gender and location – that is provided by reporters, since narrative text is harder to obtain and process. We applied a clinical Natural Language Processing (NLP) system with demonstrated performance on adverse event reports to extract certain clinical features, like symptoms, diagnoses and treatments, from the text for comparison.
The probabilistic approach to record linkage has been shown to be more effective than a deterministic one, in which a subset of fields must all match exactly, so it is unsurprising that other tools have been based on the Fellegi-Sunter model as well, including the vigiMatch method employed in WHO’s VigiBase system. Some have also incorporated features that have not (yet) been included in our algorithm, like vigiMatch’s ability to assign a higher ‘weight’ score to matches on rarely reported values (e.g. Country=‘Montenegro’, with only about 800 reports in the entire database) than to matches on commonly reported values (e.g. Country=‘Germany’, with over 500,000 reports). Enhancements like these will undoubtedly lead to improvement in our model, but the greatest growth potential resides in properly capturing the information from narratives to allow automated expert-level comparison between case reports.
Although the inclusion of features from the case narrative text did not generally improve the automated classification performance of our algorithm in this particular study, we remain confident that narratives provide valuable benefits. We have twice demonstrated that a deduplication algorithm utilizing free text can return high-quality candidate lists of potential duplicate cases for expert review. One was in this study, where we found that 11 of the 14 highest ranked pairs in a data set were true duplicates (with the 4 other true duplicates ranked at lower levels). The other instance was an experiment that was a precursor to that work, in which we used only textual information and patient sex to identify a set of 53 clusters of 2-4 reports that appeared to be duplicated; subsequent expert review confirmed 49 of them.
Further, narratives contain a considerable amount of information that may be missing from structured fields, because it is either not recorded in the appropriate place(s) or not feasible to encode at all. The latter may include facets like medical or family history, or temporal information about the progression of symptoms. We will continue to refine the NLP tool for capturing this information because unlocking the potential of free text narratives will benefit a litany of public health and clinical data issues, not just deduplication.
Our comments are an informal communication and represent our own best judgment. These comments do not bind or obligate FDA.
Duplicate Cases – Two or more records describing the same occurrence of events – same adverse effect(s) experienced with the same medical product at the same time – for the same patient. Due to reporting inaccuracies, two records can be considered duplicates even if they do not contain an identical list of events.
Decision Support Environment – An interactive software platform providing data and analytical tools to medical experts to facilitate decision-making.
Clinical Natural Language Processing – The automated processing of unstructured, human-written textual information from a clinical/medical setting to provide useful insight or analysis.
Read more:
- Kreimeyer K et al, “Using probabilistic record linkage of structured and unstructured data to identify duplicate cases in spontaneous adverse event reporting systems”, Drug Safety, 2017.