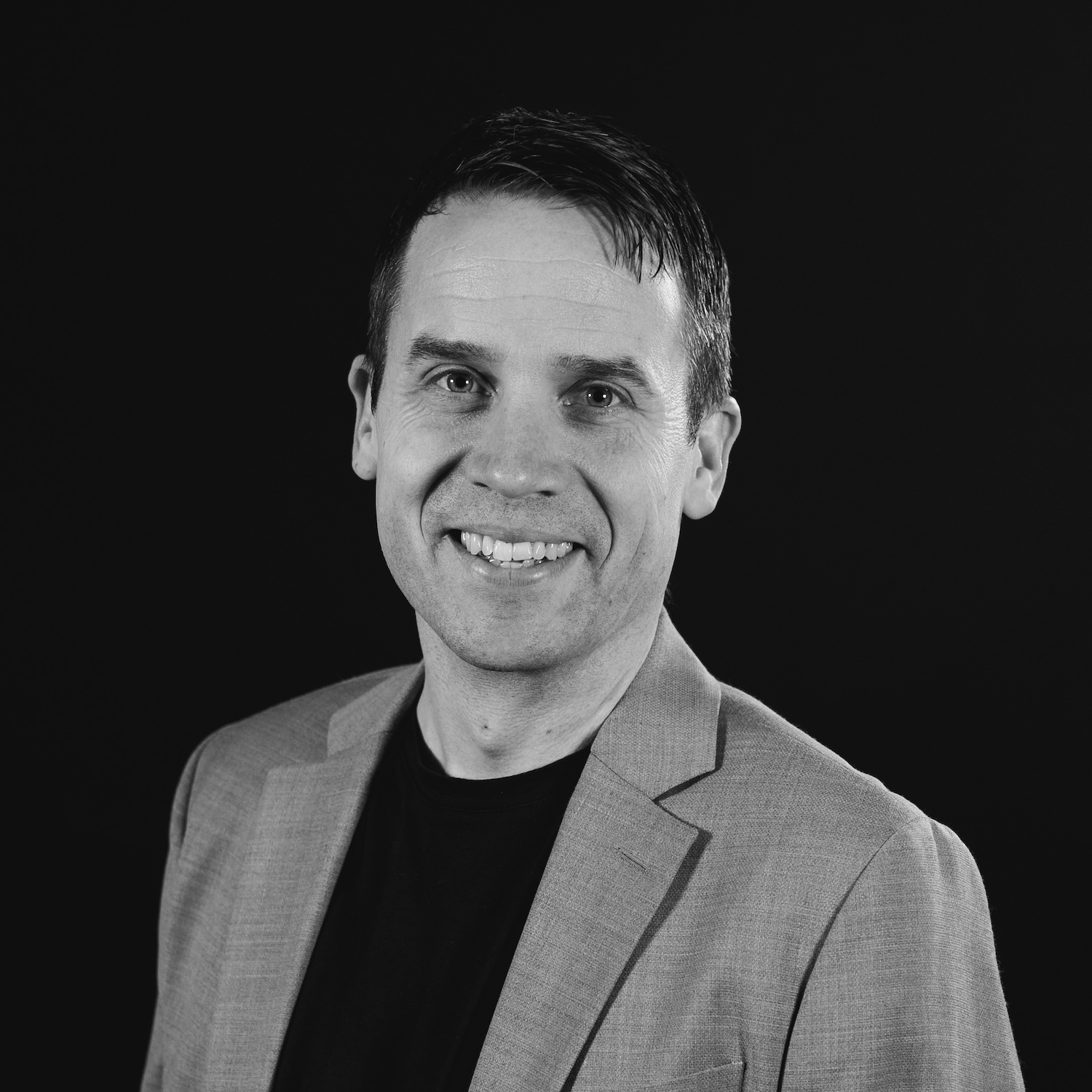
There is rapidly growing interest in the value that artificial intelligence (AI) solutions can bring to the field of pharmacovigilance and to society at large. Several contributions in this issue of Uppsala Reports reflect this. Michael Glaser and colleagues from GSK suggest that we need to look for ways to build trust in AI and machine learning solutions without sharing (or being able to interpret) their detailed parameters or code - or the data on which they were trained or evaluated. Our visiting scientist, Michele Fusaroli from University of Bologna, argues for the use of more sophisticated machine learning methods to capture and provide further insight regarding complex adverse events such as impulse control disorders. Michele’s research using network models is adapted from statistical physics, but has a similar aim as UMC’s methods for adverse event cluster analysis and semantic representations of adverse events and medicinal products. In a separate piece, UMC senior data scientist Jim Barrett describes the obstacles that case report duplication presents to effective analyses of pharmacovigilance data and our on-going efforts to refine and further improve our duplicate detection method vigiMatch.
Duplicate detection is one of the earliest examples I’m aware of where a more complex AI solution was applied at scale to improve routine pharmacovigilance operations. However, in this and other earlier scientific publications we tended to use terms like pattern discovery, machine learning, or predictive modelling instead of AI. The latter seemed poorly defined and possibly misleading, implying more general capabilities and agency than offered by the narrow applications we were working on. However, with a more precise and inclusive definition such as the one proposed by Prof Jeffrey Aronson, the term AI becomes more relevant:
artificial intelligence n. A branch of computer science that involves the ability of a machine, typically a computer, to emulate specific aspects of human behaviour and to deal with tasks that are normally regarded as primarily proceeding from human cerebral activity.
Assuming human behaviour does not need to be unique to our species but includes behaviours also exhibited by other animals, AI thus includes any application of machine learning and natural language processing, as well as expert systems that do not use machine learning but have been explicitly programmed to perform specific tasks, like IBM’s Deep Blue chess-playing computer which beat world champion Garry Kasparov in 1997.
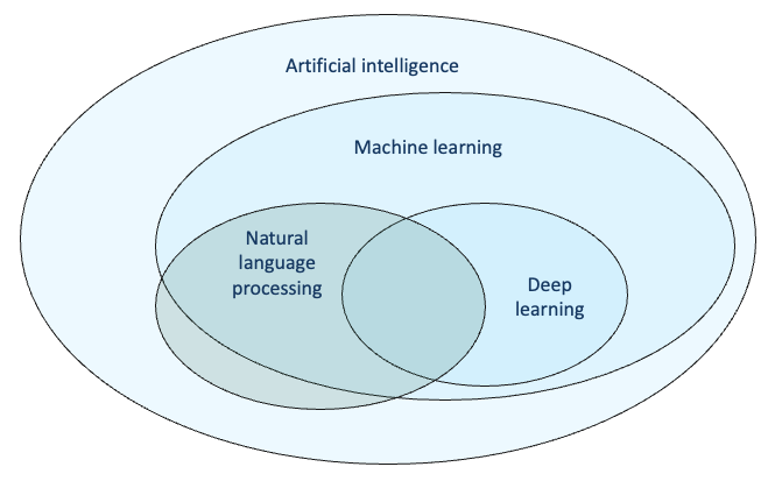
With this view, examples of AI applications in pharmacovigilance include the use of disproportionality analysis to triage drug-event combinations with subsequent expansions to drug-drug interactions and risk factors, syndrome detection, predictive models for statistical signal detection and case triaging, as well as natural language processing of regulatory information, scientific literature and case narratives, and more.
In general, when we use AI, we aim to achieve one or more of the following goals:
- Efficiency: doing the same thing faster, with less effort
- Quality: doing the same thing better, more accurately, and completely
- Capability: doing new things that were not possible before
Often, this may not be achieved by the AI solution alone, but by a human-AI team. Then, our aim is not intelligent automation but intelligence augmentation – to support and enhance human decision-making.
Generative AI brings a new level of performance to many tasks, with neural networks of unprecedented scale and so-called zero-shot learning, which allows AI solutions to perform a wide range of tasks without prior training. Generative AI may offer drastically improved performance for some existing applications and help automate or augment more complex tasks that were previously out of scope. However, it will not always be the appropriate approach. For some tasks, large language models of sufficient complexity fine-tuned for a specific task may perform better and be easier to integrate into existing workflows. For large-scale applications like database-wide duplicate detection, generative AI will likely be too slow and costly (the deployed version of vigiMatch processes 50 million report pairs per second), making traditional machine learning better suited to perform the task. In sensitive tasks like case triaging, generative AI may struggle to meet demands for reproducibility and interpretability (though so may human decision-making processes).
The ability to critically assess AI solutions will remain important and become ever more relevant to broader professional groups, including pharmacovigilance practitioners and decision-makers. CIOMS Working Group XIV on artificial intelligence in pharmacovigilance brings together experts from regulatory authorities, pharmaceutical industry, and academia to develop guidance on this topic. If we get this right, I believe that it will help improve our success rate and reduce costly errors in future investments to develop or acquire AI solutions for pharmacovigilance, ultimately contributing to the safer use of medicines and vaccines for everyone, everywhere.
Read More
M Glaser, “How do we ensure trust in AI/ML when used in pharmacovigilance?”, Uppsala Reports, 2024.
M Fusaroli, “Updating our approach to study adverse reactions”, Uppsala Reports, 2024.
J Barrett, “Weeding out duplicates to better detect side-effects”, Uppsala Reports, 2024.
Working Group XIV – Artificial Intelligence in Pharmacovigilance –