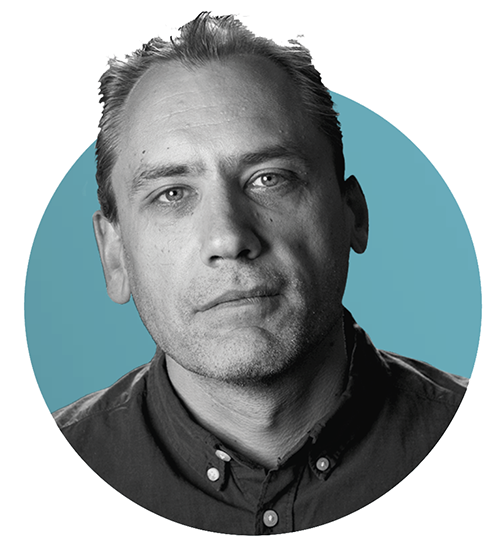
Originally developed to code medications that are not being studied but which a patient is taking during clinical trials in addition to the drug under investigation, WHODrug Koda may have found a new application – coding drugs on spontaneous reports used to monitor and assess the safety of medicines.
A paper published today in a special issue of Drug Safety looking at the role of artificial intelligence and machine learning in pharmacovigilance – "Automated Drug Coding Using AI: An Evaluation of WHODrug Koda on Adverse Event Reports" – shows UMC’s coding engine achieves the same level of automation and quality in drug coding of adverse event reports as for clinical trial data (read the Novo Nordisk study into concomitant drugs in clinical trials here), potentially making it a game changer in both drug development and post-marketing surveillance.
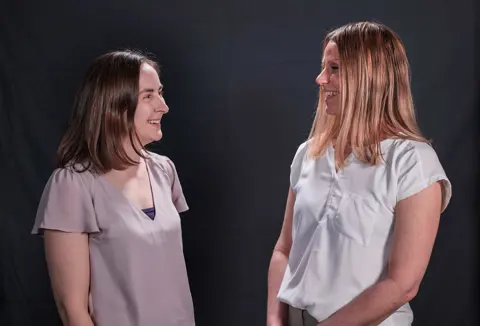
Koda’s predictions were tested against a dataset of 1,936,062 adverse event reports already coded by UMC in WHO’s global database. For the purposes of the study UMC used a direct match text algorithm as a comparator to code verbatims (drug names reported in free text) to the correct record in WHODrug Global. Koda was found to increase the level of automation from 61% to 89% while achieving the same coding quality as existing coding practices in 97% of cases.
This is no mean feat – especially when you factor in that spontaneous reports typically contain less information than clinical trial reports. In those reports key differentiators such as route and indication can inform decision-making in more challenging cases where the trade name is not enough to code a drug correctly.
“Even with potentially lower quality data, Koda performs very well despite being trained and developed for clinical trials where it typically has more information to go on,” says one of the paper’s authors, Emma Rofors. “This shows it can automate coding even when information is missing.”
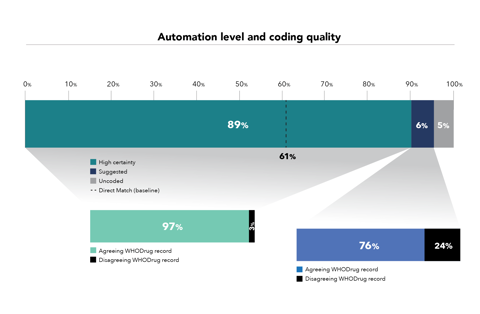
UMC CURRENTLY uses a combination of text-processing algorithms and a specially compiled synonym list to directly match the verbatims in adverse event reports to the correct WHODrug record. But synonym lists and text processing methods alone are not enough to confirm nonspecific or ambiguous drug names with certainty.
When verbatims contain abbreviations, misspellings, or ambiguous trade names that might not match directly to a record in WHODrug, trained experts need to step in and make coding decisions. “This is very time consuming,” says lead author Eva-Lisa Meldau. “The big difference with Koda is that it doesn’t just rely on verbatim information. It can also use additional information such as route, indication and country to match to a record in WHODrug, enabling automatic coding of ambiguous drug names.”
This is done through continuously training Koda to recognise and adjust to different data based on UMC know-how and industry best practice.
“At the most basic or simplistic level – direct matching the verbatim to WHODrug Global – the absolute difference in automated coding with Koda is 28%,” Rofors says. “We suspect companies are more sophisticated in their automated coding processes than this baseline suggests. They probably have their own coding dictionary based on their own synonym list, but that requires a significant amount of upkeep. Koda can be trained to do that for them.”
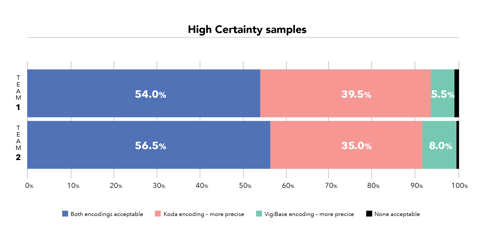
Not only is Koda more efficient, but the quality of its coding was also found to be the same or better when compared with the WHODrug entries on adverse event reports in WHO’s database. “In terms of quality we saw that in more than 50% of cases encodings were as ‘acceptable’. But in the other cases we felt Koda was coding more precisely to a more specific record in WHODrug than what was in the database,” Meldau says.
And it is consistent, which is a perennial challenge in drug coding. If you have a difficult case, Koda will always give you the same prediction. This makes it easier for teams that are dispersed across various locations to maintain coding consistency. “When you’re consistent it’s easier to achieve high-quality coding results and it will speed up the review process as well,” Rofors says. “Fixed rules based on industry best practice are built into Koda and updated continuously. We also retrain Koda twice a year so that it gets better and learns from its mistakes.”
Read more
E-L Meldau, S Bista, E Rofors, L Gattepaille, “Automated drug coding using AI: An evaluation of WHODrug Koda on adverse event reports”, Drug Safety, Volume 45, Issue 5, May 2022
S Herrgard et al, “Assessment of Machine Learning Methods in Coding of Concomitant Medications in Clinical Trials”, Novo Nordisk, 2020
WHODrug Koda – the coding engine
For more information contact WHODrug@who-umc.org